A substantial corpus of research has shown that occupancy-related factors, such as presence/absence and movement of occupants, significantly influence energy use and the indoor environmental quality in buildings. Targeting reliable occupancy information is,therefore, a key to achieving efficient HVAC system operations and building management systems, as they are designed to maintain occupants’ comfort.
Occupancy prediction has been recognized as one of the most inspiring topics for energy saving in buildings. Different approaches have been used for occupancy prediction, such as statistical models or a combination of statistical and physical models. Another common approach that is state-of-the-art in this context is deep learning. However, typical deep neural networks, which are deterministic models, do not provide any information on the uncertainty of predictions and predict a value confidently. Although studies that have used deterministic deep learning sometimes show an acceptable accuracy, it can be seen that the exact number of occupants is not predicted most of the time. Therefore, in such highly stochastic applications, deep learning models can be further enhanced by providing information about how the model is certain about its prediction. The objective of this paper is, therefore, to investigate the use of probabilistic deep learning in the context of occupancy prediction, which is overlooked so far.
Download sourceShare this
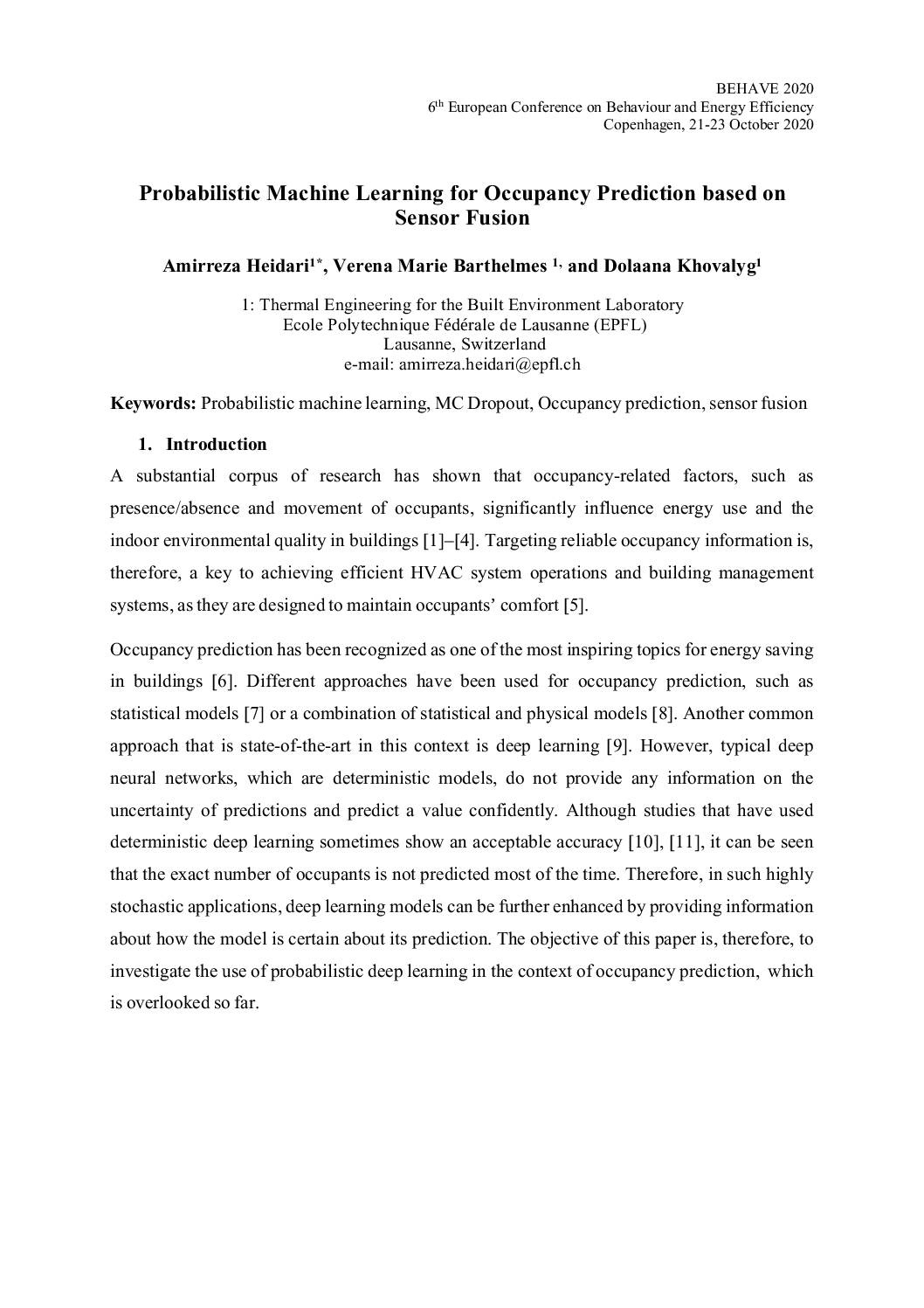
Sectors: Cross cutting, Digital
Country / Region: Global
Tags: Common Approach, decision making under uncertainty, energy, energy savings, HVAC, paper production, statistical models, sustainable livelihoods approaches, targets, uncertaintyIn 1 user collection: Session 3a: Digital Solutions for Behaviour Change
Knowledge Object: Publication / Report
Author: Amirreza Heidari, Verena Marie Barthelmes, and Dolaana Khovalyg
Content: